The Role of Emotion AI in Analyzing Open-Ended Survey Responses
- bhaveshmane
- Feb 10
- 3 min read
Updated: Feb 24
In the digital age, surveys have become a powerful tool for gathering customer insights, employee feedback, and public opinions. While closed-ended questions provide structured data that is easy to quantify, open-ended survey responses offer a wealth of qualitative insights. However, the unstructured nature of these responses presents a significant challenge. Emotion AI, also known as affective computing, has emerged as a transformative technology to address this challenge. By leveraging machine learning and natural language processing (NLP), Emotion AI can analyze and interpret the emotions conveyed in open-ended survey responses, delivering deeper and more actionable insights.
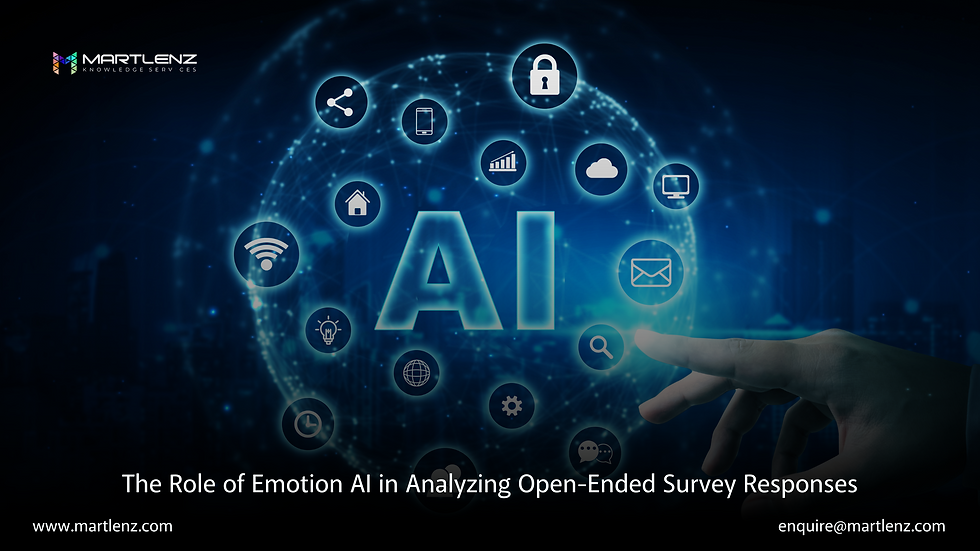
Why Open-Ended Responses Matter
Open-ended survey questions allow respondents to express themselves in their own words, offering context and nuance that are often missing in closed-ended responses. For example, while a Net Promoter Score (NPS) question can tell you how likely someone is to recommend a product, an open-ended follow-up question can reveal the "why" behind their score.
These responses are invaluable for:
Identifying emerging trends: New concerns, preferences, or opportunities often surface in open-ended feedback.
Uncovering emotional drivers: Understanding the feelings behind a response provides context to customer satisfaction or dissatisfaction.
Enhancing decision-making: Rich, qualitative data complements quantitative insights, enabling more informed strategies.
However, the sheer volume of text data from open-ended responses can make manual analysis impractical. This is where Emotion AI steps in.
How Emotion AI Works
Emotion AI combines advanced NLP techniques with machine learning algorithms to analyze unstructured text and detect emotional tone, sentiment, and intent. Here are the key components:
Text Preprocessing: The AI cleans and organizes raw text data by removing noise such as typos, irrelevant words, and stop words.
Sentiment Analysis: The system evaluates whether the sentiment expressed is positive, negative, or neutral. For instance, "The new feature is amazing" reflects a positive sentiment, while "The app keeps crashing" indicates a negative one.
Emotion Detection: Going beyond sentiment, Emotion AI identifies specific emotions such as happiness, frustration, anger, or excitement. This adds depth to the analysis, revealing the "emotional color" of responses.
Topic Modeling: AI clusters similar responses and identifies recurring themes or topics within the data.
Contextual Understanding: Advanced models, such as those based on transformers (e.g., GPT), understand the context in which words are used, ensuring more accurate interpretations.
Benefits of Using Emotion AI
Scalability: Emotion AI can process thousands of responses in a fraction of the time it would take a human analyst, making it ideal for large-scale surveys.
Accuracy and Consistency: Human analysts can introduce bias or fatigue into the process. Emotion AI ensures consistent evaluation across all responses.
Deeper Insights: By identifying emotions and sentiments, the technology uncovers layers of meaning that might be missed in a manual review.
Actionable Outcomes: Insights derived from Emotion AI can inform targeted actions, such as improving customer support, refining products, or addressing employee concerns.
Real-World Applications
Customer Feedback: Companies use Emotion AI to analyze product reviews and customer service surveys. For example, an e-commerce platform can pinpoint frustrations related to delivery times or website usability.
Employee Engagement: HR teams leverage Emotion AI to gauge employee morale from open-ended survey responses, identifying underlying issues affecting workplace satisfaction.
Market Research: Emotion AI helps marketers understand how consumers perceive their brand, products, or advertising campaigns.
Healthcare: In patient surveys, Emotion AI can detect concerns or anxieties, enabling healthcare providers to offer better support.
Challenges and Considerations
While Emotion AI offers transformative potential, there are challenges to be mindful of:
Cultural Nuances: Emotions and expressions can vary across cultures. Models need to be trained on diverse datasets to ensure accuracy.
Data Privacy: Processing sensitive feedback requires strict adherence to data privacy regulations like GDPR.
Interpretation Complexity: Emotions are complex and multifaceted. A single response may express mixed emotions, requiring careful interpretation.
The Future of Emotion AI in Survey Analysis
As Emotion AI continues to evolve, its capabilities will expand further. Advancements in NLP and machine learning will enable even more nuanced understanding of human emotions. Future applications may include real-time feedback analysis, integration with video and voice data, and predictive modeling to anticipate trends based on emotional patterns.
Conclusion
Emotion AI is revolutionizing the way organizations analyze open-ended survey responses. By transforming unstructured text into meaningful insights, it empowers businesses to understand and act on the emotions of their customers, employees, and stakeholders. In an era where emotional intelligence is increasingly valued, leveraging Emotion AI is not just a technological advancement—it is a strategic necessity.
Commentaires